In the Search of AI for Oncology
By Cagatay Culcuoglu MBA, Chief Technology Officer, Massive Bio Inc, and Arturo Loaiza-Bonilla MD MSEd, Chief Medical Officer, Massive Bio Inc, and Vice Chairman of Medical Oncology, Cancer Treatment Centers of America
Several industries have been transforming by the implementation of new technologies. Healthcare has already joined this race of introducing technology supported value to key players in its own domain: patients, doctors, clinics, pharmaceuticals, insurance companies, CROs, biotech companies. Oncology, the study and treatment of tumors, can be brought to next level in 3 major areas by exploring benefits of Artificial Intelligence, machine learning, automation and blockchain: Diagnosis, Decision Making & Treatment, Research.
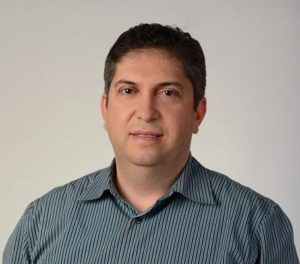
Diagnosis
With HIPAA rules in place, patient and healthcare data cannot be shared efficiently across healthcare stakeholders. However, the Office of the National Coordinator for Health Information Technology (ONC) proposes to implement the “information blocking” prohibition of the 21st Century Cures Act which can alleviate roadblocks for transparent data share. In addition, new healthcare blockchain solutions that are emerging can solve data in transit and at rest security concerns. Still, with 23.6 million new cancer cases per year globally by 2030, this is not an answer to build efficiency with vast amount of cancer patient data: scans, radiology results, next-generation sequencing files, immunohistochemistry results, pathology analysis, lab reports, treatment information, drugs that are required to diagnose a patient. Reviewing, entering, and analyzing all this valuable data set, is an enormous uptake for nurses, and doctors.
Now by applying cognitive technologies medical information, treatment options, clinical trials can be reviewed and stored faster than any human. Machine learning can support to manage all this critical mix of data in a usable format and can help interpret the diagnosis of a patient. Unstructured data is other the part of the puzzle. Lack of standardization and rule sets across institutions result in more data integration and preparation issues to kick start AI efforts. Although, federal government has issued standards for the electronic health record (EHR) and formats such as FHIR for health care data exchange, published by HL7 seem to be in place, unexpected data gaps for basic patient information can be observed at clinical institutions due to operational inefficiencies, or lack of expertise and interest in having a robust internal clinical data.
Decision Making and Treatment
Timeliness and accuracy are key in decision making and in building a personalized treatment plan for cancer patients. Using pattern recognition in machine learning with supported or unsupported models to identify patients’ condition for probable treatment options based on improved clinical pathways or matching them to clinical trials is where we observe happening more frequently.
A more comprehensive approach for disease management, better coordinate care plans and help patients to better manage and comply with their long-term treatment roadmaps is in demand but can only be achieved with technology and clinical expertise moving along hand in hand. These implementations at hospital level integrated with EMRs would alleviate the pressure on staff and doctors, improve patient satisfaction by identifying less toxic, better performing systemic therapy solutions commingled with on the spot genomic recommendations and probably improve progression-free survival rates with decreased costs. Understanding clinical pathways, leveraging existing ontologies can speed up implementation of these AI initiatives.
Research
On average it will cost a company to develop a new cancer drug from the research lab to the patient is $650 million and it takes at least ten years for a new cancer cure to be on the shelves, with clinical trials alone taking at least 4 years. Regardless a randomized or a non-randomized clinical trial such as TAPUR which is open and enrolling patients at 113 sites, a clinical part of reach and development efforts might involve all types of drugs into account as inclusion or exclusion criteria or for intervention: chemotherapy, immunotherapy, targeted drugs applied standalone or in combination as regimens. Here, AI can be applied to drug research and discovery by streamlining the drug discovery, increased clinical trial recruitment, drug phase repurposing, population densities. Results would be observed as time to market improvement, decreased drug development costs.
For healthcare professionals, one of the main challenges is around trusting on the AI or in other words black box problem of AI. A mistake at anywhere in the chain of AI solution might result in an unwanted situation for patients. But it is possible to overcome these concerns.
In the adoption of this set of AI technologies, building a team around technological know-how is a good start but not enough. On the side of technologists who are experts on natural language processing, neural networks, variations of machine learning methodologies, phyton or R, you would need to build a multidisciplinary team with doctors, nurses, genomics experts, data scientists. Rigorous tests and validating AI based outputs by these experts would ensure more reliable results. This would have to be supported with real-world data and patient outcomes and be part of the loop to feedback into machine learning algorithms. This leads to one probable issue – too much trust in the system, as humans we get comfortable with ease. It would be wise to have a guardian of AI, reviewing the output of the algorithm at a non-stop pace.
Healthcare and cancer treatment is on the brink of change in the next 10 years thanks to AI and other innovative technologies. However, it all depends on us as in our own corporate silos or network to work in collaboration.
Pingback: In the Search of AI for Oncology - Massive Bio