Is AI replacing radiologists?
By George Renchao Wu, Senior Diagnostic Radiology Resident, and Arash Kardan, Chief of Nuclear Medicine and Molecular Imaging, Baylor College of Medicine
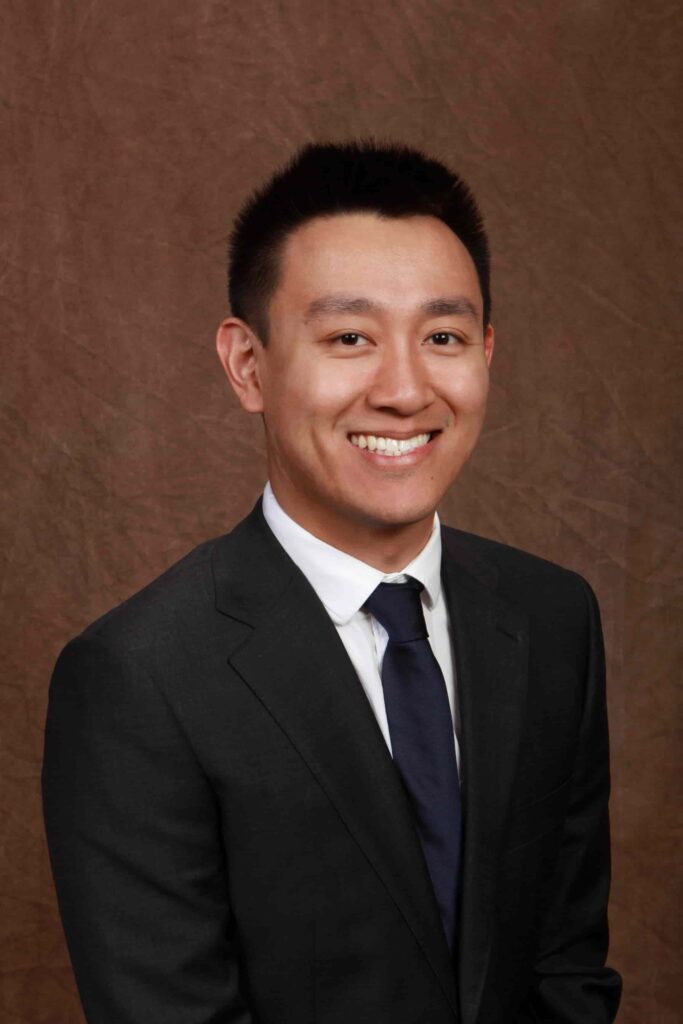
The future of healthcare is here. Will physicians be a part of it?
Flying cars, interstellar space travel, and ubiquitous sentient intelligence running megacities. The idea of a technologically advanced future has mesmerized society for as long as one can remember. Yet, the past is riddled with false predictions by futurists. As one of the more technologically forward medical specialties, radiology is at the forefront of advances in healthcare artificial intelligence (AI). However, predictions of AI’s influence on radiology have not aged well. In 2016, Dr. Geoffrey Hinton, regarded as the “godfather of deep learning,” famously stated that society should stop training radiologists, that it was obvious radiologists would be replaced by software in the next five years. Having decided on radiology as a career early on in medical school in 2014, I would be lying if I said I wasn’t a tiny bit concerned about my career choice. Since then, seven years have passed and not a single radiologist has been replaced by autonomous software. Was Dr. Hinton completely wrong about AI? Or did he simply get the timing wrong?
Computers assisting radiologists are nothing new. Computer-aided diagnosis (CAD) was approved by the USFDA in 1998 for mammography, and CMS approved reimbursement for CAD in 2002. When a radiologist reads a mammogram, CAD software highlights sinister-looking calcifications and masses for a radiologist to review. While there was some initial concern that CAD would replace radiologists, that never materialized. On the contrary, CAD was another tool in a radiologist’s armamentarium in their quest to diagnose cancer. When today’s radiologists read about the current AI landscape, many dismiss the notion that their jobs are at risk. Many believe that, like CAD in the past, new AI technology will overpromise and underdeliver. Instead, I would argue that current AI software is completely different. We are in uncharted waters.
Today’s AI software is powered by deep learning algorithms, a subset of the machine learning that utilizes artificial neural networks such as convolutional neural networks (CNN) to recognize and make sense of imaging findings with significantly less need for manual filter labeling, a necessity of more archaic algorithms that was both tedious and resource-intensive. With high-quality annotated training data, researchers are able to use these sophisticated algorithms to make sense of the mountains of information in diagnostic imaging datasets. Most importantly, they are performing some tasks with an accuracy similar to or better than practicing radiologists.
What steps need to be taken to arrive in a world where machines have replaced radiologists?
In 2017, the world was introduced to CheXNet, a CNN-powered algorithm that exceeded the performance of practicing radiologists at the task of identifying pneumonia on chest radiographs. The algorithm was trained on ChestX-ray14, a large publicly WU 1 available dataset of frontal chest radiographs, and performance was compared to four practicing radiologists. It did not take long for the media to sensationalize the news, clamoring that the end was near for radiologists. Since then, AI software has made significant inroads in nearly all subspecialties of radiology. Whether it is software that automatically calculates and compares the volume of brain cancers, or a startup that sends stroke findings directly to a neurointerventionalist’s smartphone, we are seeing the discipline of radiology being shaped right before our eyes.
As of January 2023, the FDA has cleared almost 400 radiology AI algorithms, with more than half of the algorithms on the market receiving clearance from 2019 to 2022. What is driving this surge? For one, it is helpful that the optics around AI have shifted, thanks in
part to an optimistic outlook of a future where machines help us, not hurt us. Computing power has also advanced and is less cost prohibitive. But arguably, the biggest catalyst is reimbursement. Since CMS implemented new payment models for AI software, the
market has exploded with startups. Stroke software developed by companies have circumvented the diagnostic radiologist completely. These algorithms automatically review images to quickly identify patients who would benefit from immediate neurovascular intervention. If this software is used in a center where only a single radiologist is interpreting studies for the entire hospital, it’s not uncommon for the interventionalist to know which patients need treatment before the radiologist knows the exam has even been performed. Best of all? Hospitals using these algorithms can be reimbursed by CMS under a new pathway called New Technology Add-On Payment (NTAP), allowing for quick and widespread adoption.
Yet, we are nowhere near Dr. Hinton’s predicted reality. Where do we go from here? What steps need to be taken to arrive in a world where machines have replaced radiologists? For starters, the public needs to be comfortable with the idea of a machine telling them they have brain cancer. The financial incentives need to be there to allow competition to foster innovation. Above all, algorithms need to grow more sophisticated. It’s one thing to perform a singular task better than a radiologist. It’s something else entirely to replace an entire discipline of medicine.
Amara’s Law states that society tends to overestimate the impact of technology in the short term and underestimate in the long term. We are seeing this play out now. Despite all the growth we’ve seen in the short term, there are moments of obvious disconnect between reality and expectations. In a world marching towards automation, it is paramount that humanity remains at the heart of medicine. For all the things that computers excel at, being human is something it can never achieve. It is imperative that physicians serve as gatekeepers, to ensure that patients do not suffer at the hands of those who prioritize profit over care.